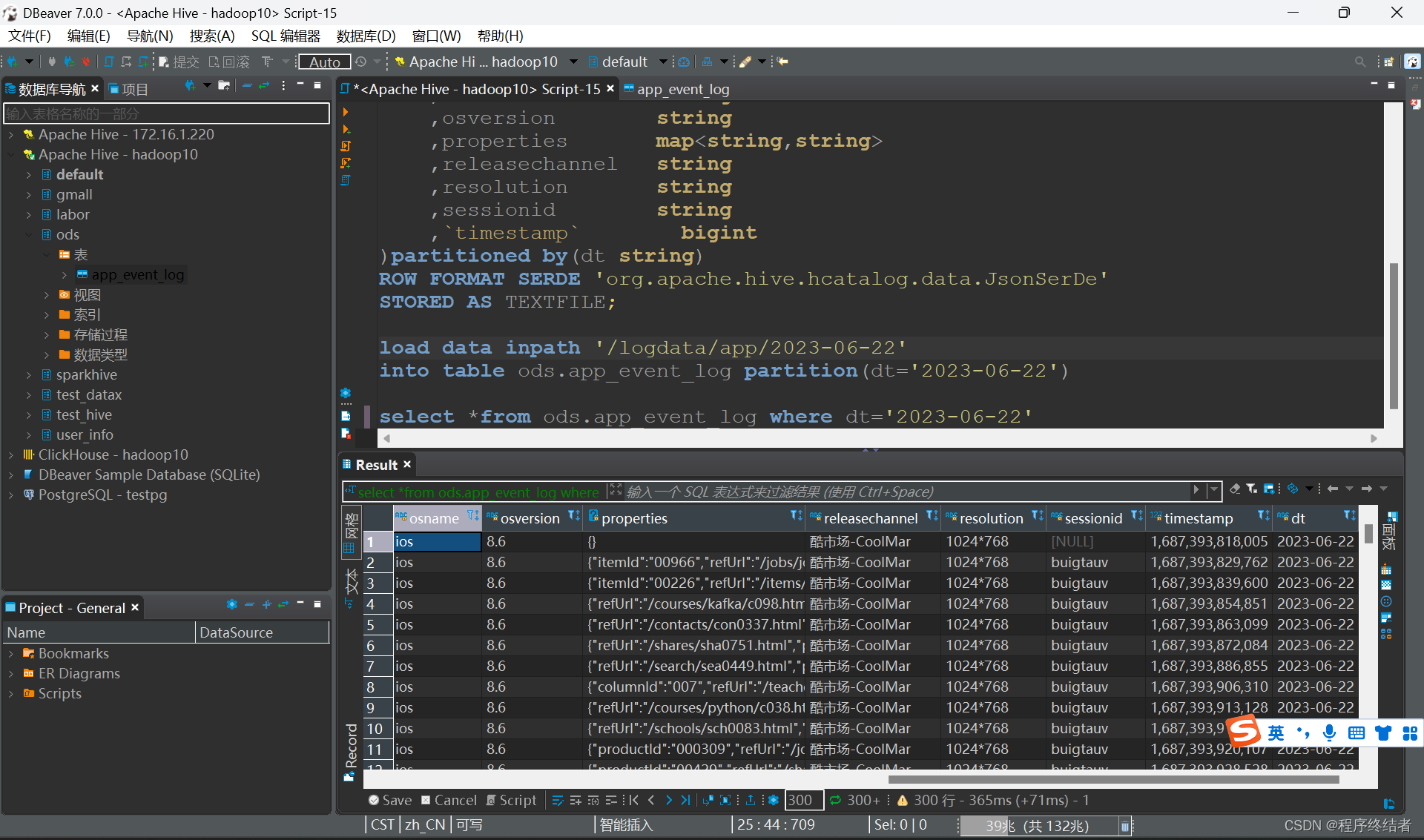
【Spark数仓项目】需求一:项目用户数据生成-ODS层导入-DWD层初步构建
本项目使用一台虚拟机,主机名Hadoop10,CentOS7系统。虚拟机服务器jdk选用1.8,包括完整生态的hadoop spark;本地主机为win11,scala2.12.17,在idea集成环境中编写。
文章目录
写在前面:
本项目需求运行在CentOS7下Hadoop10单机环境:
- Spark3.2.0
- Flink1.13.6
- Hadoop3.1.4
- jdk1.8
- Sqoop1.4.6
- MySQL5.7
- Hive3.1.2
- Kafka0.11
- Flume1.9.0
- Zookeeper3.4.6
- Hbase2.4
- Redis6.2.0
- Dlink0.7.3
Windows11 开发环境:
- Idea 2020
- Moba
- DBeaver7.0.0
- Scala2.12.17
本项目使用一台linux虚拟机,主机名Hadoop10,CentOS7系统。
虚拟机服务器jdk选用1.8,包括完整生态的hadoop spark;
本地主机为win11,scala2.12.17,在idea集成环境中编写。
一、项目数据准备工作
sh脚本执行jar生成用户数据和日志行为数据:“/opt/app/genlog.sh”
jar文件位置:“/opt/app/log-generator-jar-with-dependencies.jar”
1.1 生成测试数据代码指令:
[root@hadoop10 app]# sh genlog.sh user
generating user data ...
[root@hadoop10 app]# sh genlog.sh log
generating log data ...
1.2 生成用户和行为数据位置:
上传到HDFS
这里上传2023-06-21的日期,以后的需求中需要更多的日期数据,需要重复进行该小节的步骤生成数据。请注意,由于本次的课程项目是在模拟实际生产环境,因此直到上传到HDFS才可以算作是T-1日的log数据完成。
[root@hadoop10 sparkdw]# hdfs dfs -mkdir -p /logdata/app/2023-06-21
[root@hadoop10 sparkdw]# hdfs dfs -put /root/moni_data/app.access.log.2023-06-21 /logdata/app/2023-06-21
二、Hive导入ODS层数据
本节内容将ODS层数据完成构建在Hive数据库中,Hive提前启动。本节提供Hive数据库的搭建,ODS层代码,以及多种导入数据的方式,包括使用Shell脚本导入数据库的方式,使用Shell脚本是为了方便程序能够上调度。
2.1 ODS层建表语句:
create database ods;
create table ods.app_event_log(
account string
,appid string
,appversion string
,carrier string
,deviceid string
,devicetype string
,eventid string
,ip string
,latitude double
,longitude double
,nettype string
,osname string
,osversion string
,properties map<string,string>
,releasechannel string
,resolution string
,sessionid string
,`timestamp` bigint
)partitioned by(dt string)
ROW FORMAT SERDE 'org.apache.hive.hcatalog.data.JsonSerDe'
STORED AS TEXTFILE;
load data inpath '/logdata/app/2023-06-22'
into table ods.app_event_log partition(dt='2023-06-22')
select *from ods.app_event_log where dt='2023-06-22'
方式一:直接在Hive中导入HDFS中的原始日志数据,导入成功截图:
2.2 执行导入脚本
这是方拾贰:
使用shell脚本"01.导入数据到ODS.sh":
#! /bin/bash
#可以传递一个日期参数,如果没有写就使用T-1日期
#sh 01.导入数据到ODS.sh 2023-06-22
#load data inpath '/logdata/app/2023-06-22' into table ods.app_event_log partition(dt='2023-06-22')
#sh 01.导入数据到ODS.sh
#load data inpath '/logdata/app/2023-06-25' into table ods.app_event_log partition(dt='2023-06-25')
dt=$1
if [ "x"$1 == "x" ]
then
dt=$(date -d "1 days ago" +%Y-%m-%d)
fi
echo " 执行日期 ---------- $dt ----------------- "
#name=zs
#echo "姓名:'$name'"
#echo '姓名:"$name"'
#hive -e "sql"
hive -e "load data inpath '/logdata/app/$dt' into table ods.app_event_log partition(dt='$dt')"
此脚本的好处是可以将生成日志日期给一个变量指定。
[root@hadoop10 sparkdw]# sh 01.导入数据到ODS.sh 2023-06-21
三、DWD层数据清洗
DWD层将进行初步的数据清洗。由于数据清洗是经常性的,每天都要执行的代码应该写入shell脚本,本章小节会使用shell脚本调用scala程序,将jar包放在服务器上使用yarn模式运行,在spark上进行数据清洗工作。其中编写了两个Spark工具类,用于测试和生产环境中更方便的读取配置文件和传日期变量。
3.1 Hive建库语句:
开始数据库insert操作之前需要在hive中创建临时表,如下:
create database tmp;
create table if not exists tmp.event_log_washed(
account string
,appid string
,appversion string
,carrier string
,deviceid string
,devicetype string
,eventid string
,ip string
,latitude double
,longitude double
,nettype string
,osname string
,osversion string
,properties map<string,string>
,releasechannel string
,resolution string
,sessionid string
,`timestamp` bigint
)partitioned by(dt string)
STORED AS orc
TBLPROPERTIES ('orc.compress'='SNAPPY');
select * from tmp.event_log_washed;
3.2 Spark本地模式测试:
数据清洗代码编写开始之前需要新建一个maven项目,项目名见下面代码。
3.2.1 maven依赖管理
注意:此依赖是本章节所有项目的完整依赖,包括本地模式和优化后的服务器提交yarn模式。
<?xml version="1.0" encoding="UTF-8"?>
<project xmlns="http://maven.apache.org/POM/4.0.0"
xmlns:xsi="http://www.w3.org/2001/XMLSchema-instance"
xsi:schemaLocation="http://maven.apache.org/POM/4.0.0 http://maven.apache.org/xsd/maven-4.0.0.xsd">
<modelVersion>4.0.0</modelVersion>
<groupId>org.example</groupId>
<artifactId>spark-dw</artifactId>
<version>1.0-SNAPSHOT</version>
<dependencies>
<dependency>
<groupId>org.apache.spark</groupId>
<artifactId>spark-core_2.12</artifactId>
<version>3.2.0</version>
</dependency>
<dependency>
<groupId>mysql</groupId>
<artifactId>mysql-connector-java</artifactId>
<version>5.1.38</version>
</dependency>
<dependency>
<groupId>org.apache.spark</groupId>
<artifactId>spark-sql_2.12</artifactId>
<version>3.2.0</version>
</dependency>
<dependency>
<groupId>org.apache.spark</groupId>
<artifactId>spark-hive_2.12</artifactId>
<version>3.2.0</version>
</dependency>
<dependency>
<groupId>org.apache.hive.hcatalog</groupId>
<artifactId>hive-hcatalog-core</artifactId>
<version>3.1.2</version>
</dependency>
<dependency>
<groupId>com.fasterxml.jackson.core</groupId>
<artifactId>jackson-annotations</artifactId>
<version>2.12.1</version>
</dependency>
<dependency>
<groupId>com.fasterxml.jackson.core</groupId>
<artifactId>jackson-core</artifactId>
<version>2.12.4</version>
</dependency>
<dependency>
<groupId>com.fasterxml.jackson.core</groupId>
<artifactId>jackson-databind</artifactId>
<version>2.12.4</version>
</dependency>
<dependency>
<groupId>com.typesafe</groupId>
<artifactId>config</artifactId>
<version>1.3.3</version>
</dependency>
</dependencies>
<build>
<finalName>spark-dw</finalName>
<extensions>
<extension>
<groupId>org.apache.maven.wagon</groupId>
<artifactId>wagon-ssh</artifactId>
<version>2.8</version>
</extension>
</extensions>
<plugins>
<plugin>
<groupId>net.alchim31.maven</groupId>
<artifactId>scala-maven-plugin</artifactId>
<version>4.4.0</version>
<executions>
<execution>
<id>scala-compile-first</id>
<phase>process-resources</phase>
<goals>
<goal>add-source</goal>
<goal>compile</goal>
</goals>
</execution>
</executions>
</plugin>
<plugin>
<artifactId>maven-assembly-plugin </artifactId>
<configuration>
<descriptorRefs>
<descriptorRef>jar-with-dependencies</descriptorRef>
</descriptorRefs>
</configuration>
<executions>
<execution>
<id>make-assembly</id>
<phase>package</phase>
<goals>
<goal>single</goal>
</goals>
</execution>
</executions>
</plugin>
<plugin>
<groupId>org.codehaus.mojo</groupId>
<artifactId>wagon-maven-plugin</artifactId>
<version>1.0</version>
<configuration>
<!--上传的本地jar的位置-->
<fromFile>target/${project.build.finalName}.jar</fromFile>
<!--远程拷贝的地址-->
<url>scp://root:root@hadoop10:/opt/app</url>
</configuration>
</plugin>
</plugins>
</build>
</project>
在spark scala编写数据清洗代码:
还有一个resources目录下的配置文件:hive-site,这一节忘了给了,在下一小节中给出了,是关于hive元数据的。
package com.yh.ods_etl
import org.apache.spark.sql.SparkSession
object AppLogWash_01 {
def main(args: Array[String]): Unit = {
val spark = SparkSession.builder()
.master("local[*]")
.appName("AppLogWash_01")
.enableHiveSupport().getOrCreate();
/**
* 过滤掉日志中缺少关键字段(deviceid/properties/eventid/sessionid 缺任何一个都不行)的记录
* 过滤掉日志中不符合时间段的记录(由于app上报日志可能的延迟,有数据延迟到达)
* ods.app_event_log
* tmp.event_log_washed
*/
spark.sql(
"""
|
|insert overwrite table tmp.event_log_washed
|partition(dt='2023-06-22')
|select
| account
| ,appid
| ,appversion
| ,carrier
| ,deviceid
| ,devicetype
| ,eventid
| ,ip
| ,latitude
| ,longitude
| ,nettype
| ,osname
| ,osversion
| ,properties
| ,releasechannel
| ,resolution
| ,sessionid
| ,`timestamp`
|from ods.app_event_log
|where dt = '2023-06-22'
|and deviceid is not null and trim(deviceid) != ''
|and eventid is not null and trim(eventid) != ''
|and sessionid is not null and trim(sessionid) != ''
|and properties is not null and size(properties) > 0
|and from_unixtime(cast(substring(`timestamp`,1,10) as bigint),'yyyy-MM-dd') = '2023-06-22'
|
|""".stripMargin)
spark.stop()
}
}
3.2.2 本地模式运行成功截图:
此为在spark中的local模式下运行,仅用于测试环境,在生产环境中需要打包进服务器。
运行spark scala代码后查看hive表:
3.3 服务器提交yarn模式:
开始之前需要将刚才local模式中插入的数据清空,以便于测试:
select * from tmp.event_log_washed where dt='2023-06-22'
alter table tmp.event_log_washed drop partition(dt='2023-06-22')
3.3.1 编写scala清洗文件:
AppLogWash_01.scala:
注意:这个scala文件中主函数传入了一个参数,是为了便于在服务器提交时写日期参数,如果在spoark中测试时,可以在edit configuration中填写,如图:
package com.yh.ods_etl
import com.yh.utils.SparkUtils
object AppLogWash_01 {
def main(args: Array[String]): Unit = {
if(args.length == 0){
println("缺失参数")
System.exit(0)
}
val spark = SparkUtils.getSparkSession("AppLogWash_01")//传参数APPname
/**
* 过滤掉日志中缺少关键字段(deviceid/properties/eventid/sessionid 缺任何一个都不行)的记录
* 过滤掉日志中不符合时间段的记录(由于app上报日志可能的延迟,有数据延迟到达)
* ods.app_event_log
* tmp.event_log_washed
*/
val dt = args(0)
spark.sql(
s"""
|
|insert overwrite table tmp.event_log_washed
|partition(dt='${dt}')
|select
| account
| ,appid
| ,appversion
| ,carrier
| ,deviceid
| ,devicetype
| ,eventid
| ,ip
| ,latitude
| ,longitude
| ,nettype
| ,osname
| ,osversion
| ,properties
| ,releasechannel
| ,resolution
| ,sessionid
| ,`timestamp`
|from ods.app_event_log
|where dt = '${dt}'
|and deviceid is not null and trim(deviceid) != ''
|and eventid is not null and trim(eventid) != ''
|and sessionid is not null and trim(sessionid) != ''
|and properties is not null and size(properties) > 0
|and from_unixtime(cast(substring(`timestamp`,1,10) as bigint),'yyyy-MM-dd') = '${dt}'
|
|""".stripMargin)
spark.stop()
}
}
3.3.2 resources目录中的三个配置文件:
这三个配置文件结合下一小节中的两个工具类食用。
application.properties:
此文件为修改local模式和yarn模式的配置开启关闭,需要手动操作。
#local.run为true代表在本地测试,否则在集群测试,打包之前改为false
local.run=false
common-version-info.properties:
version=2.7.6
hive-site.xml:
<?xml version="1.0" encoding="UTF-8" standalone="no"?>
<?xml-stylesheet type="text/xsl" href="configuration.xsl"?>
<configuration>
<!--
mysql数据库在hive体系架构中的作用? 答案:存储元数据
元数据:描述数据的数据
spark整合hive开发,读取hive表需要连接mysql数据库获取元数据信息
spark(hive cli) 连接hadoop10已经启动的metastore metastore连接mysql数据库
-->
<property>
<name>hive.metastore.uris</name>
<value>thrift://hadoop10:9083</value>
</property>
</configuration>
3.3.3 utils工具类两个:
SparkUtils.scala:
切换模式yarn和local模式
package com.yh.utils
import org.apache.spark.sql.SparkSession
object SparkUtils {
def getSparkSession(appName:String) ={
var spark:SparkSession = null
if(ConfigUtils.flag){
spark = SparkSession.builder()
.master("local[*]")
.appName(appName)
.enableHiveSupport().getOrCreate()
}else{
spark = SparkSession.builder()
.master("yarn")
.appName(appName)
.enableHiveSupport().getOrCreate()
}
spark
}
}
ConfigUtils.scala:
通过配置文件确定当前模式是local还是yarn
package com.yh.utils
import com.typesafe.config.{Config, ConfigFactory}
object ConfigUtils {
//1.加载application.properties配置文件
val config: Config = ConfigFactory.load()
//2.根据key获取value
val flag = config.getBoolean("local.run")
}
3.3.4 打包jar,上传至服务器
在这里需要注意的是,经过本地测试后,上传到服务器的jar包一定要是yarn模式的,通过前面我们编写的传参主函数传入日期。jar包的名字可以通过配置finalname修改。我们所使用的依赖也需要全部打包进jar包上传到服务器,使用插件可以完成将所有依赖打包的操作。上述事项只需要复制前文中我整理好的依赖管理即可正常打包使用,完整jar包大约293M。
打包成功截图:
jar包上传到服务器
服务器提交命令:
[root@hadoop10 app]# spark-submit --master yarn --class com.yh.ods_etl.AppLogWash_01 /opt/app/spark-dw-jar-with-dependencies.jar 2023-06-22
23/06/26 06:43:46 WARN NativeCodeLoader: Unable to load native-hadoop library for your platform... using builtin-java classes where applicable
23/06/26 06:43:50 WARN Client: Neither spark.yarn.jars nor spark.yarn.archive is set, falling back to uploading libraries under SPARK_HOME.
23/06/26 06:44:27 WARN SessionState: METASTORE_FILTER_HOOK will be ignored, since hive.security.authorization.manager is set to instance of HiveAuthorizerFactory.
提交成功,查看完成的数据:
编写shell运行:
[root@hadoop10 sparkdw]# sh 02.数据清洗.sh 2023-06-22
执行日期 ---------- 2023-06-22 -----------------
23/06/26 06:52:40 WARN NativeCodeLoader: Unable to load native-hadoop library for your platform... using builtin-java classes where applicable
23/06/26 06:52:43 WARN Client: Neither spark.yarn.jars nor spark.yarn.archive is set, falling back to uploading libraries under SPARK_HOME.
23/06/26 06:53:19 WARN SessionState: METASTORE_FILTER_HOOK will be ignored, since hive.security.authorization.manager is set to instance of HiveAuthorizerFactory.
02.数据清洗.sh
#! /bin/bash
dt=$1
if [ "x"$1 == "x" ]
then
dt=$(date -d "1 days ago" +%Y-%m-%d)
fi
echo " 执行日期 ---------- $dt ----------------- "
spark-submit \
--master yarn \
--class com.yh.ods_etl.AppLogWash_01 \
--conf spark.defalut.parallelism=10 \
--driver-memory 1G \
--driver-cores 2 \
--executor-memory 2G \
--num-executors 3 \
--executor-cores 2 \
--queue abc \
/opt/app/spark-dw-jar-with-dependencies.jar $dt
3.3.5 在上一步优化一下 submit提交方式
1.解决了23/06/26 09:14:23 WARN Client: Neither spark.yarn.jars nor spark.yarn.archive is set, faploading libraries under SPARK_HOME.
这个警告问题。这个警告信息表明在提交Spark应用程序时,没有明确指定spark.yarn.jars或spark.yarn.archive参数,导致Spark将依赖的库文件上传到SPARK_HOME目录下。
2.解决了每次都要打包上传大文件依赖的问题,将--jars /opt/app/spark-dw-jar-with-dependencies.jar
进行指定。
#! /bin/bash
dt=$1
if [ "x"$1 == "x" ]
then
dt=$(date -d "1 days ago" +%Y-%m-%d)
fi
echo " 执行日期 ---------- $dt ----conf spark.defalut.parallelism=10 \--------------- "
spark-submit \
--master yarn \
--class com.yh.ods_etl.AppLogWash_01 \
--conf spark.yarn.jars=local:/opt/installs/spark3.2.0/jars/* \
--driver-memory 1G \
--driver-cores 2 \
--executor-memory 2G \
--num-executors 3 \
--executor-cores 2 \
--queue abc \
--jars /opt/app/spark-dw-jar-with-dependencies.jar \
/opt/app/spark-dw.jar $dt
更多推荐
所有评论(0)