sklearn K折(KFold)交叉验证案例,展开细节代码与cross_validate简写
文章目录一、通常的随机森林模型代码二、K折交叉验证的随机森林代码1. 切分方式:随机切分2.切分方式:不均衡数据集下按比例切分三、KFold的简便写法四、随机森林预测与KFold交叉验证完整代码一、通常的随机森林模型代码对于一个基本的随机森林预测模型:from sklearn.ensemble import RandomForestClassifierimport pandas as pdimpo
一、通常的随机森林模型代码
对于一个基本的随机森林预测模型:
from sklearn.ensemble import RandomForestClassifier
import pandas as pd
import numpy as np
from sklearn.metrics import accuracy_score
def get_train_x_y():
from sklearn.preprocessing import StandardScaler
x = pd.DataFrame(data=np.random.randint(0, 10, size=(1000, 5)))
y = np.random.randint(0, 2, 1000)
x = StandardScaler().fit_transform(x)
return x, y
if __name__ == '__main__':
x_train, y_train = get_train_x_y()
# 切分训练集与测试集
from sklearn.model_selection import train_test_split
x_train, x_test, y_train, y_test = train_test_split(x_train, y_train, test_size=0.3)
# 使用随机森林预测
my_model = RandomForestClassifier(n_estimators=10)
my_model.fit(x_train, y_train)
# 预测并得到准确率
result_prediction = my_model.predict(x_test)
score = accuracy_score(y_test, result_prediction)
print(score) # 得到预测结果区间[0,1]
二、K折交叉验证的随机森林代码
1. 切分方式:随机切分
使用K折前,前面都不变,从main函数开始:
if __name__ == '__main__':
x_train, y_train = get_train_x_y()
# 切分训练集与测试集,注意所有的交叉验证等都是在训练集上做的操作,测试集只有最后的最后才会使用到
from sklearn.model_selection import train_test_split
x_train, x_test, y_train, y_test = train_test_split(x_train, y_train, test_size=0.3)
# 使用随机森林建模
my_model = RandomForestClassifier(n_estimators=10)
然后就是设置KFold,n_splits=5
时,切分训练集为5组
# 使用交叉验证
from sklearn.model_selection import KFold
kfold = KFold(n_splits=5)
for train_index, test_index in kfold.split(x_train, y_train):
# train_index 就是分类的训练集的下标,test_index 就是分配的验证集的下标
this_train_x, this_train_y = x_train[train_index], y_train[train_index] # 本组训练集
this_test_x, this_test_y = x_train[test_index], y_train[test_index] # 本组验证集
# 训练本组的数据,并计算准确率
my_model.fit(this_train_x, this_train_y)
prediction = my_model.predict(this_test_x)
score = accuracy_score(this_test_y, prediction)
print(score) # 得到预测结果区间[0,1]
之后可以把每一组的score都保存起来,计算平均方差什么的。
KFold更多参数请参考:https://scikit-learn.org/stable/modules/generated/sklearn.model_selection.KFold.html
2.切分方式:不均衡数据集下按比例切分
当然切分可以选择不同的切分方式,比如不均衡的数据集:
from sklearn.model_selection import StratifiedKFold
kfold = StratifiedKFold(n_splits=5)
这个会先将y分层,然后按照与训练集同样的比例进行切分
更多参数请参考:https://scikit-learn.org/stable/modules/generated/sklearn.model_selection.StratifiedKFold.html
三、KFold的简便写法
不需要把kfold
切分开,只需要用一个cross_validate
工具就行:
# 使用交叉验证
from sklearn.model_selection import KFold
kfold = KFold(n_splits=5)
# 使用K-fold的简写方式
from sklearn.model_selection import cross_validate
# 参数依次是:模型,训练集x,训练集y,kfold,评价指标
cv_cross = cross_validate(my_model, x_train, y_train, cv=kfold, scoring=('accuracy', 'f1'))
cv_cross
会返回每一轮的训练与预测时间,还有评价指标值,可以大大减少代码量
更多scoring
请参考:https://scikit-learn.org/stable/modules/model_evaluation.html#the-scoring-parameter-defining-model-evaluation-rules
四、随机森林预测与KFold交叉验证完整代码
from sklearn.ensemble import RandomForestClassifier
import pandas as pd
import numpy as np
def get_train_x_y():
from sklearn.preprocessing import StandardScaler
x = pd.DataFrame(data=np.random.randint(0, 10, size=(1000, 5)))
y = np.random.randint(0, 2, 1000)
x = StandardScaler().fit_transform(x)
return x, y
if __name__ == '__main__':
x_train, y_train = get_train_x_y()
# 切分训练集与测试集,注意所有的交叉验证等都是在训练集上做的操作,测试集只有最后的最后才会使用到
from sklearn.model_selection import train_test_split
x_train, x_test, y_train, y_test = train_test_split(x_train, y_train, test_size=0.3)
# 使用随机森林建模
my_model = RandomForestClassifier(n_estimators=10)
# 使用交叉验证
from sklearn.model_selection import KFold
kfold = KFold(n_splits=5)
# 使用K-fold的简写方式
from sklearn.model_selection import cross_validate
cv_cross = cross_validate(my_model, x_train, y_train, cv=kfold, scoring=('accuracy', 'f1'))
更多推荐
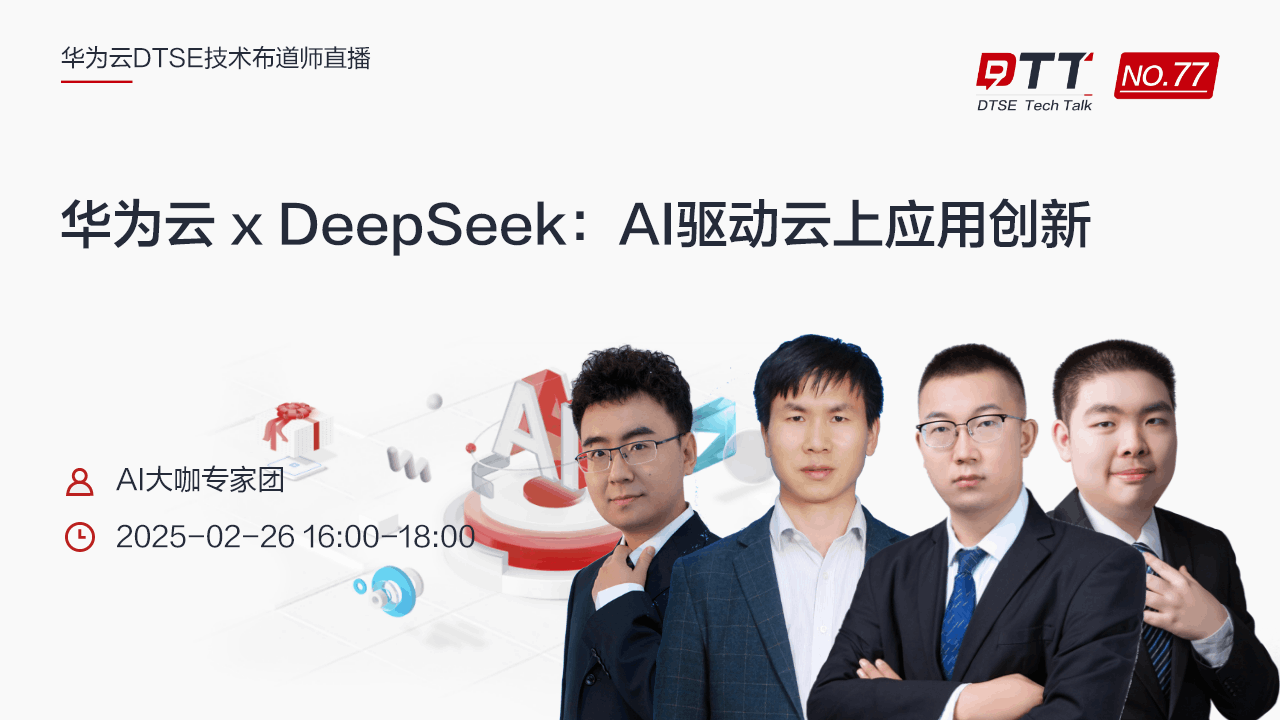

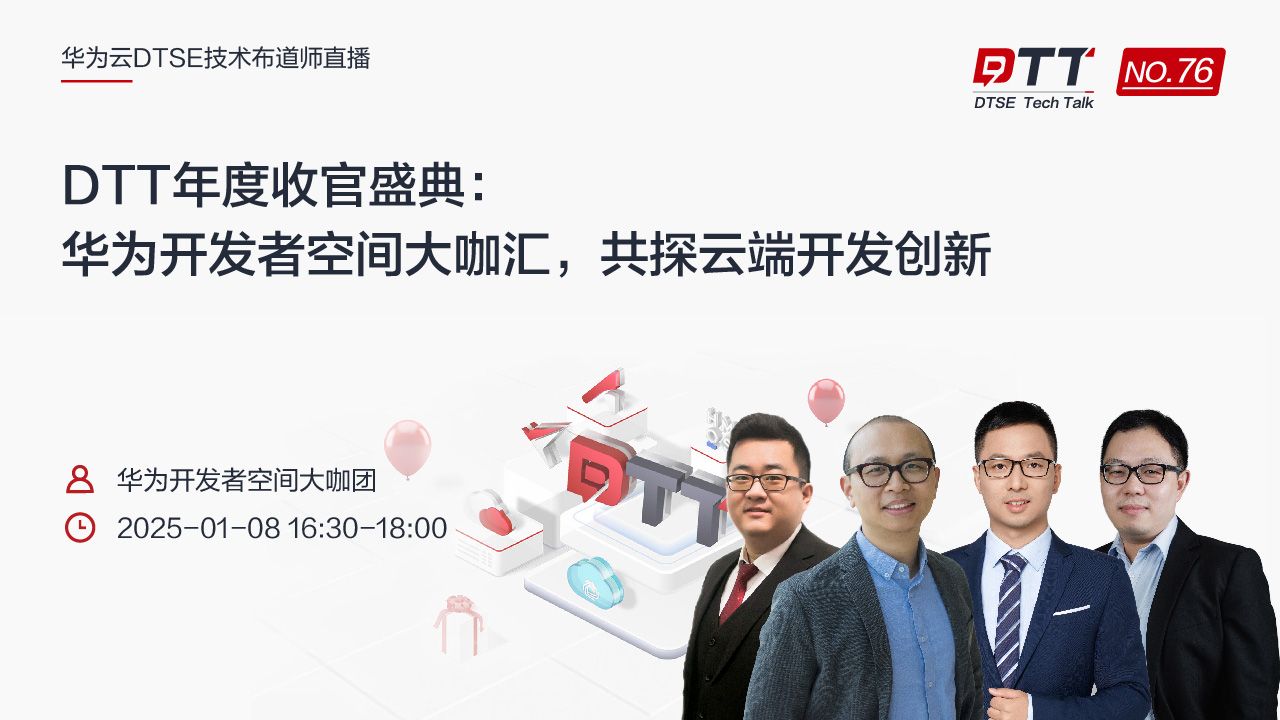

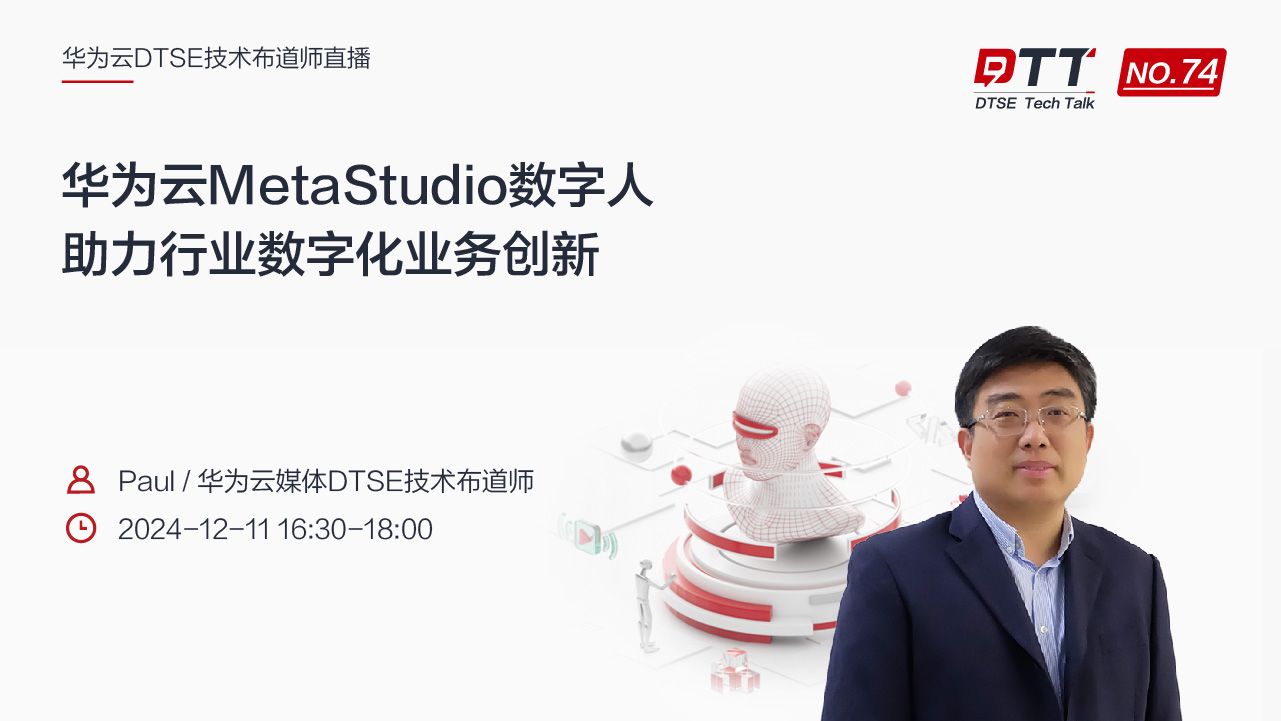

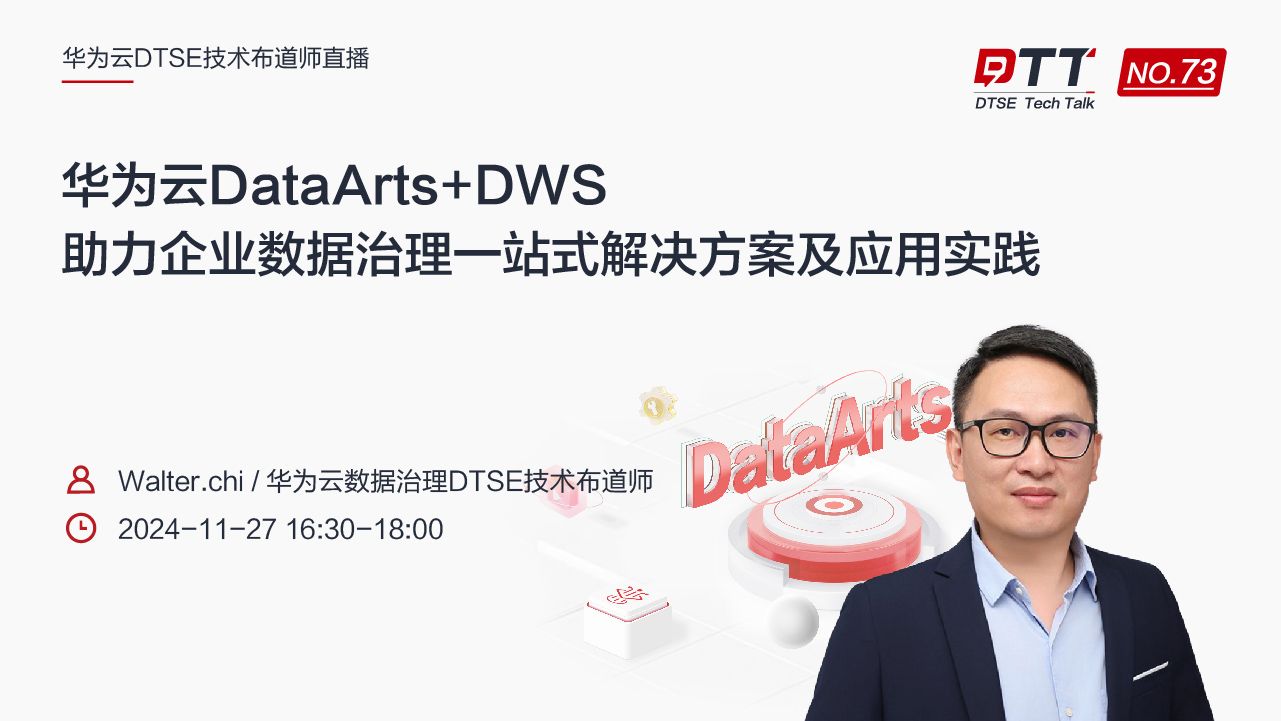

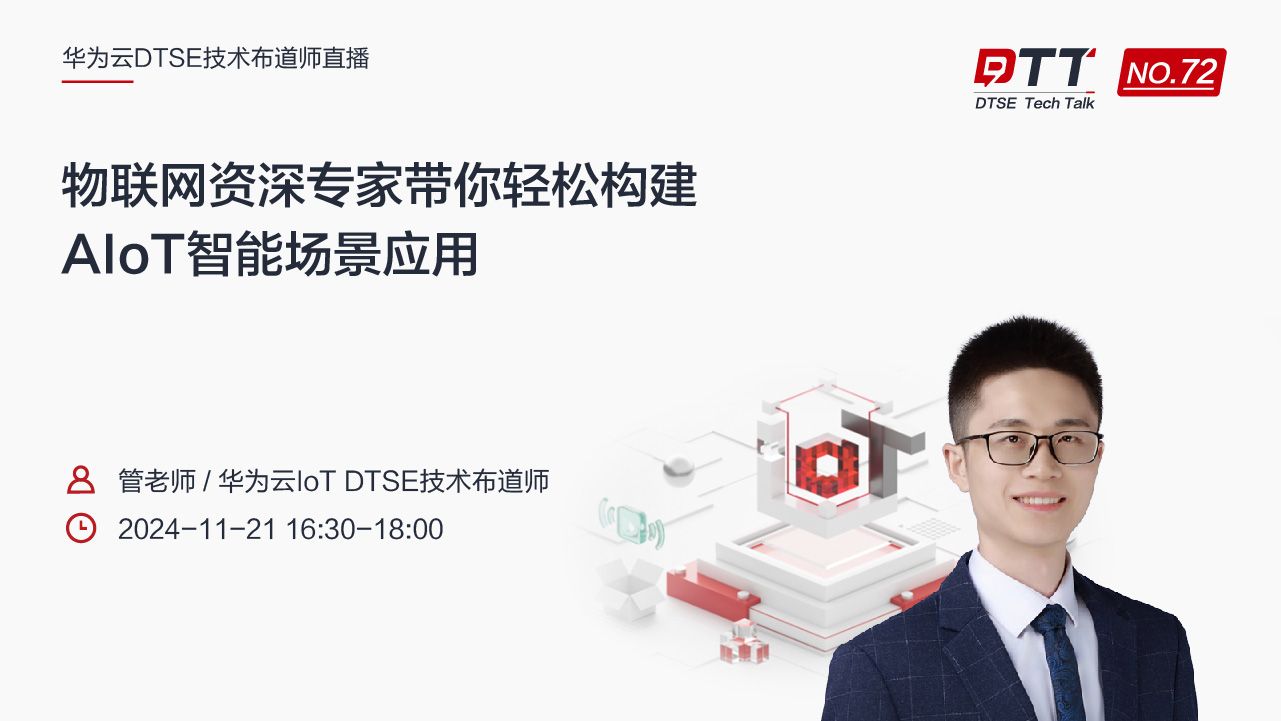

所有评论(0)