Python建立线性回归模型进行房价预测
本文讲解了Python建立线性回归模型进行房价预测的实战流程以及代码实现方法。
·
前期准备
本文使用Jupyter-notebook作为集成开发环境,使用Scikit-learn库搭建线性回归模型进行房价预测,Scikit–learn具有三大优点:
- 丰富的算法模块
- 易于安装和使用
- 样例丰富教程文档详细
官网:https://scikit-learn.org/stable/index.html
多因子房价预测
基于task2_data.csv数据,建立多因子线性回归模型
- 以面积、人均收入、平均房龄为输入变量,建立多因子模型,评估模型表现
- 预测面积=150, 人均收入=60000, 平均房龄=5的合理房价
实战流程
- 数据加载与可视化
- 数据预处理
- 建立单因子线性回归模型,训练模型
- 评估模型表现,可视化线性回归预测结果
流程图为:
1.数据加载
首先加载task2_data.csv数据并展示部分数据,代码如下:
#数据加载
import pandas as pd
import numpy as np
data = pd.read_csv('task2_data.csv')
data.head(10)
2.数据可视化
对数据进行可视化代码如下:
from matplotlib import pyplot as plt
fig = plt.figure(figsize=(20,5))
fig1 = plt.subplot(131)
plt.scatter(data.loc[:,'面积'],data.loc[:,'价格'])
plt.title('Price VS Size')
fig2 = plt.subplot(132)
plt.scatter(data.loc[:,'人均收入'],data.loc[:,'价格'])
plt.title('Price VS Income')
fig3 = plt.subplot(133)
plt.scatter(data.loc[:,'平均房龄'],data.loc[:,'价格'])
plt.title('Price VS House_age')
plt.show()
面积与价格、人均收入与价格、平均房龄与价格散点图如下:
3.数据预处理
将上述加载的数据转化为numpy格式
#X y再次赋值
X = data.drop(['价格'],axis=1)
y = data.loc[:,'价格']
X.head()
#数据预处理
X = np.array(X)
y = np.array(y)
y = y.reshape(-1,1)
print(X.shape,y.shape)
4.模型建立与训练
建立多因子回归模型 并且训练
#建立多因子回归模型 并且训练
from sklearn.linear_model import LinearRegression
model_multi = LinearRegression()
model_multi.fit(X,y)
5.模型预测
进行多因子模型的预测
#多因子模型的预测
y_predict_multi = model_multi.predict(X)
print(y_predict_multi)
6.模型评估
通过计算模型准确率与r2_score对模型进行评估
from sklearn.metrics import mean_squared_error,r2_score
MSE_multi = mean_squared_error(y,y_predict_multi)
R2_multi = r2_score(y,y_predict_multi)
print(MSE_multi)
print(R2_multi)
7.房价预测
最后预测面积=150, 人均收入=60000, 平均房龄=5的合理房价
#预测面积=150, 人均收入=60000, 平均房龄=5的合理房价
X_test = np.array([[150,60000,5]])
y_test_predict = model_multi.predict(X_test)
print(y_test_predict)
数据与代码
房价数据与完整代码github地址:https://github.com/15009824714/House-price-forecast
点击阅读全文
更多推荐
活动日历
查看更多
直播时间 2025-02-26 16:00:00
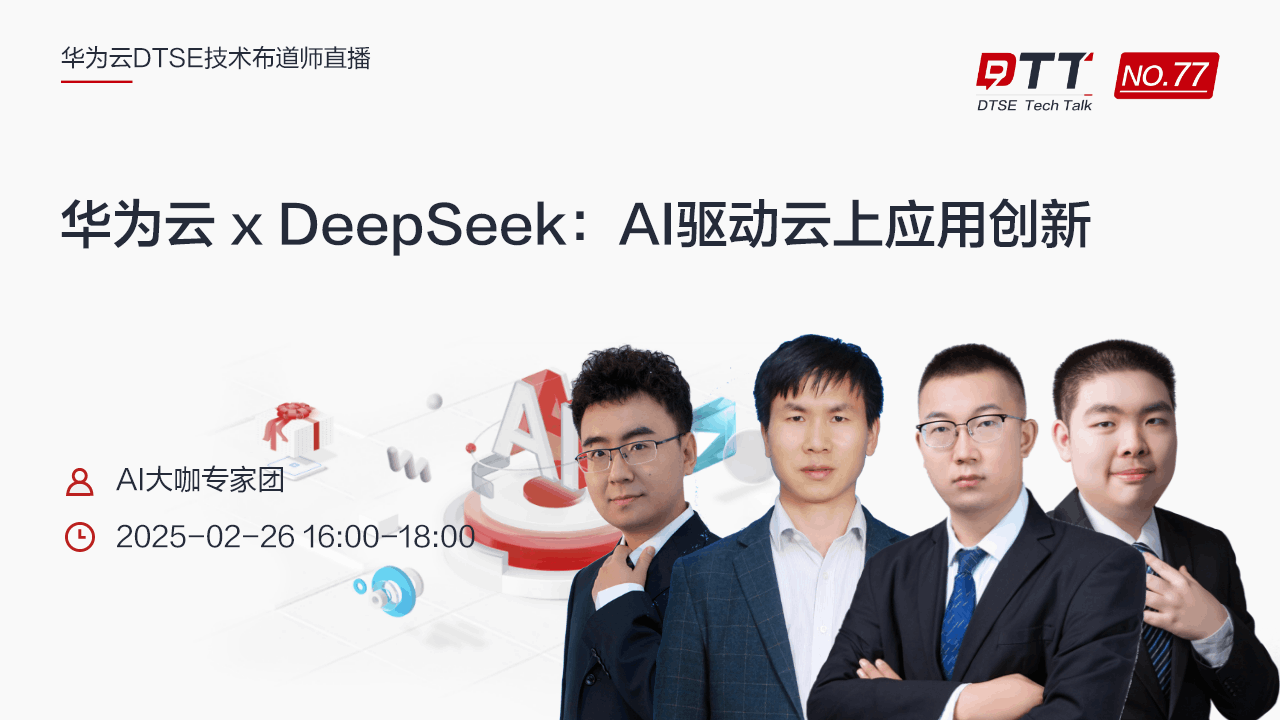

直播时间 2025-01-08 16:30:00
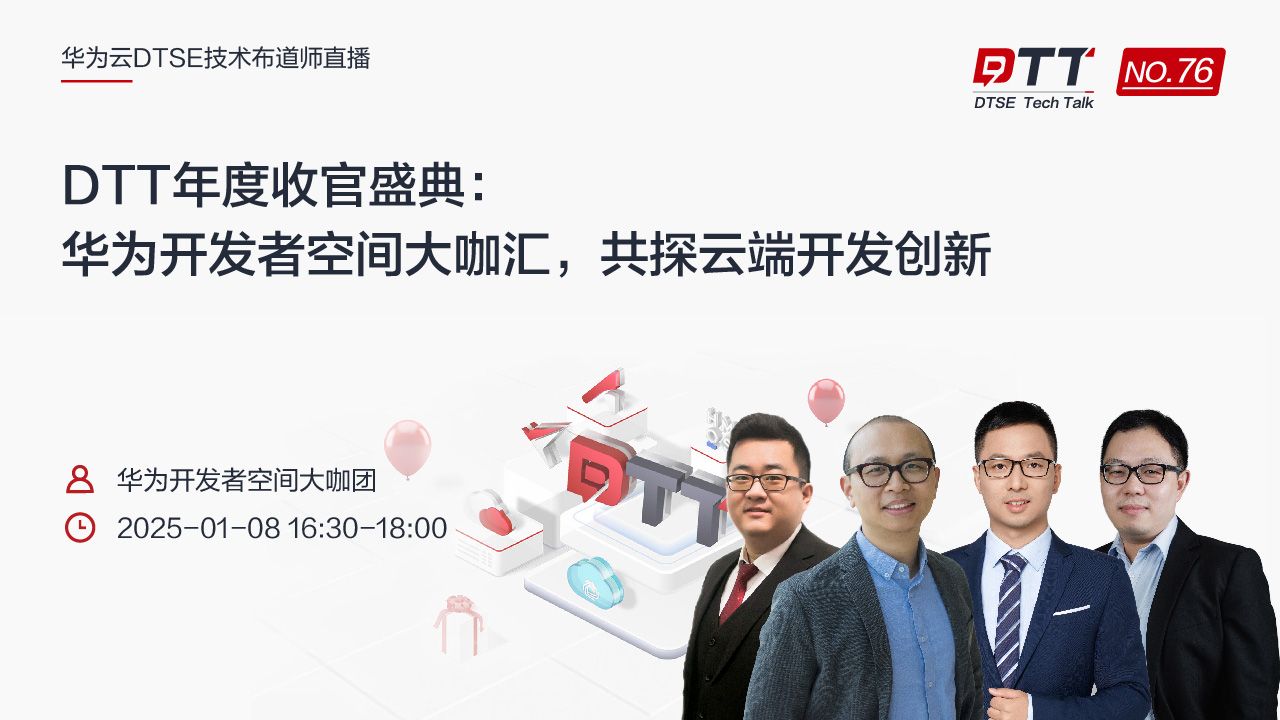

直播时间 2024-12-11 16:30:00
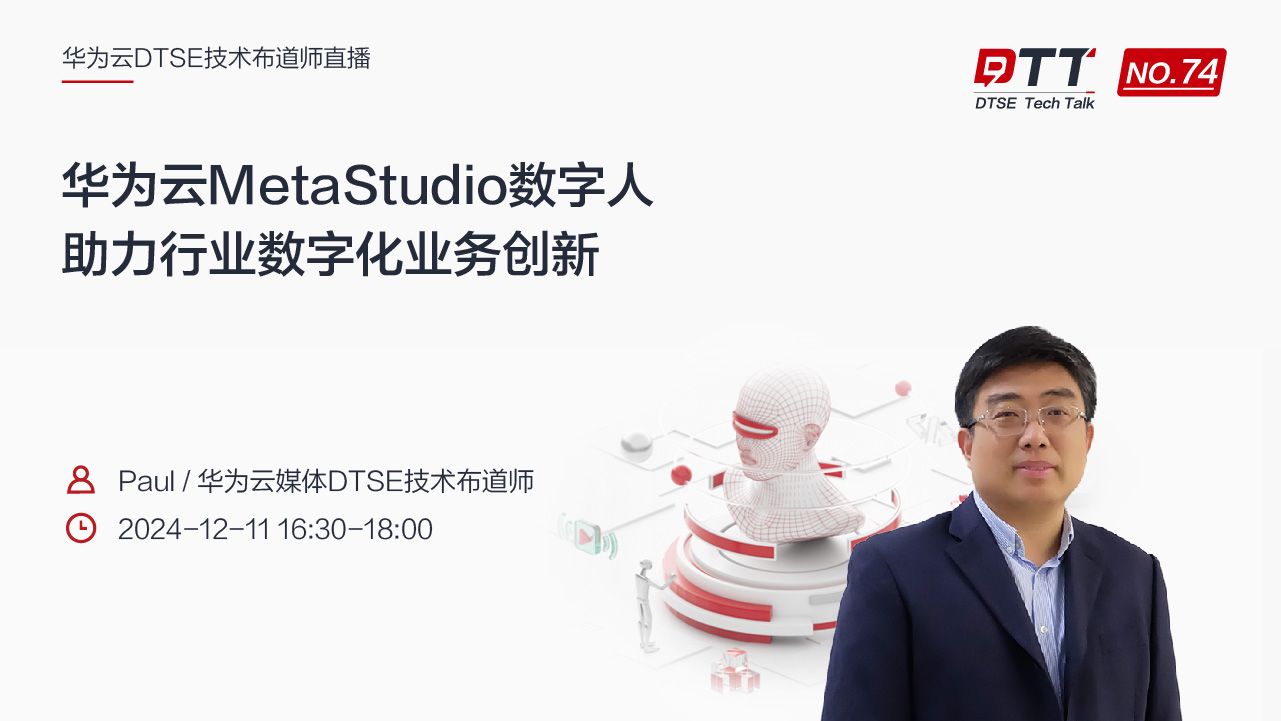

直播时间 2024-11-27 16:30:00
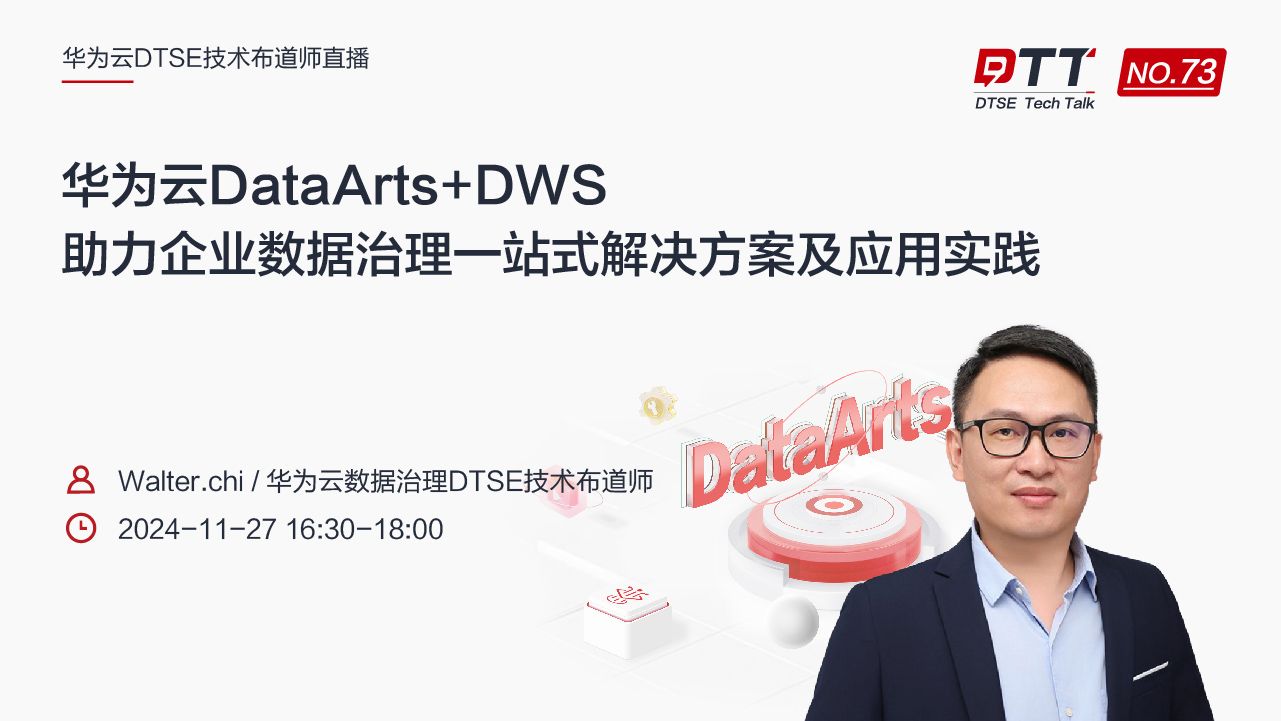

直播时间 2024-11-21 16:30:00
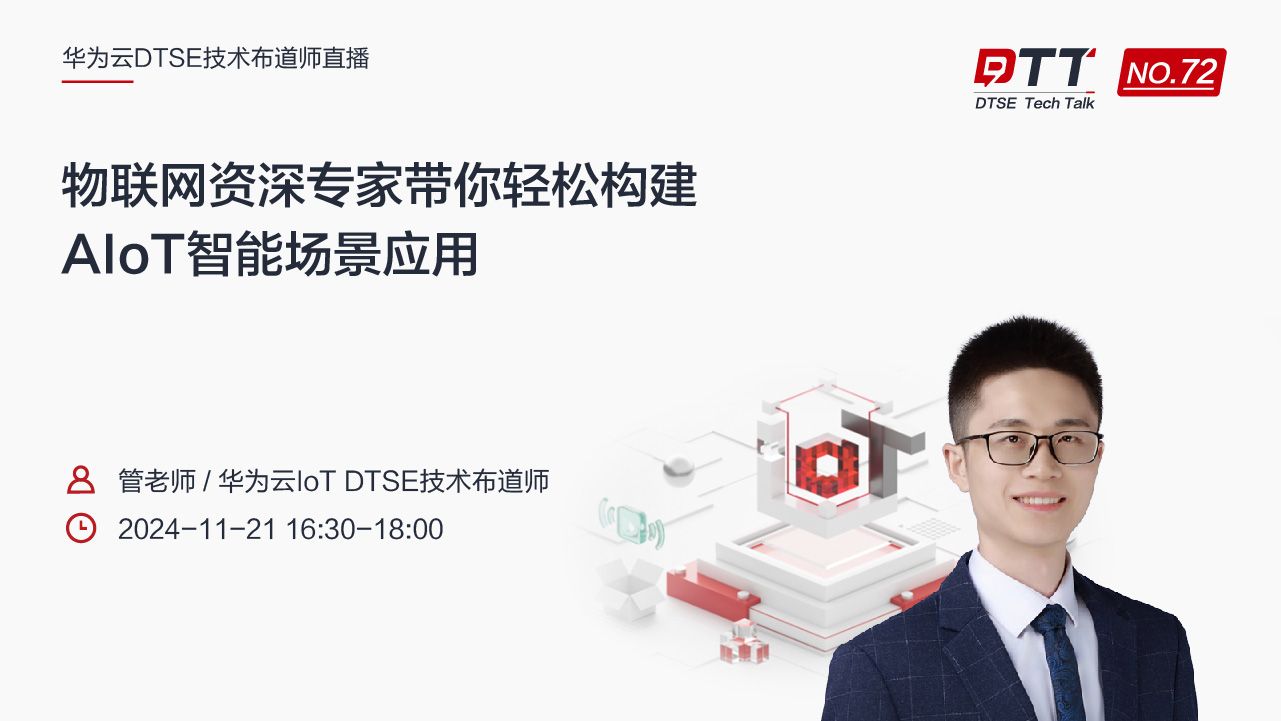

目录
所有评论(0)