【机器学习】聚类方法实例——使用K-means算法实现数据聚类分析
【机器学习】聚类方法实例——使用K-means算法实现数据聚类分析一、聚类目标1.K-Means(K均值)聚类算法步骤:优点:缺点:二、使用步骤1.生成数据2.使用K-means算法进行聚类3.评价聚类结果4.用silhouette选择K-means聚类的簇数:5.使用不同聚类方法完整代码总结参考一、聚类目标簇内相似度高,且簇间相似度低(高内聚,低耦合)(high-intra-class,low-
·
【机器学习】聚类方法实例——使用K-means算法实现数据聚类分析
一、聚类目标
簇内相似度高,且簇间相似度低(高内聚,低耦合)(high-intra-class,low-inter-class)
1.K-Means(K均值)聚类
算法步骤:
(1) 首先我们选择一些类/组,并随机初始化它们各自的中心点。中心点是与每个数据点向量长度相同的位置。这需要我们提前预知类的数量(即中心点的数量)。
(2) 计算每个数据点到中心点的距离,数据点距离哪个中心点最近就划分到哪一类中。
(3) 计算每一类中中心点作为新的中心点。
(4) 重复以上步骤,直到每一类中心在每次迭代后变化不大为止。也可以多次随机初始化中心点,然后选择运行结果最好的一个。
优点:
速度快,计算简便
缺点:
必须提前知道数据有多少类/组。
K-Medians是K-Means的一种变体,是用数据集的中位数而不是均值来计算数据的中心点。
K-Medians的优势是使用中位数来计算中心点不受异常值的影响;缺点是计算中位数时需要对数据集中的数据进行排序,速度相对于K-Means较慢。
二、使用步骤
1.生成数据
#导入需要的库
from numpy import unique
from numpy import where
from sklearn.datasets import make_classification
from sklearn.preprocessing import LabelEncoder, StandardScaler
from matplotlib import pyplot
# 将生成的分类数据集用图形化显示
X,y = make_classification(n_samples=5000, n_features=2,n_informative=2,
n_redundant=0,n_classes=3,n_clusters_per_class=1,
random_state=1)
# 数据集标准化操作
X = StandardScaler().fit_transform(X)
clusters_temp = unique(y)
for cluster_temp in clusters_temp:
row_ix = where(y == cluster_temp)
pyplot.scatter(X[row_ix,0],X[row_ix,1])
pyplot.show()#显示数据集分布情况
# y.shape
2.使用K-means算法进行聚类
from sklearn.cluster import KMeans
from matplotlib import pyplot
from numpy import unique
from numpy import where
from matplotlib import pyplot
model = KMeans(n_clusters=3)#聚类类别为2
yhat = model.fit_predict(X)
clusters = unique(yhat)
for cluster in clusters:
row_ix = where(yhat == cluster)
pyplot.scatter(X[row_ix,0],X[row_ix,1])
pyplot.show()
分类后是这个样子:
3.评价聚类结果
from sklearn import metrics
from sklearn.metrics import davies_bouldin_score
print("Adjusted Rand-Index: %.3f" % metrics.adjusted_rand_score(y,yhat))
print("davies bouldin index: %.3f"% metrics.davies_bouldin_score(X,yhat))
评价结果如下:
4.用silhouette选择K-means聚类的簇数:
import numpy as np
from sklearn.cluster import KMeans
from sklearn.metrics import silhouette_samples, silhouette_score
range_n_clusters = [2, 3, 4, 5, 6]
for n_clusters in range_n_clusters:
clusterer = KMeans(n_clusters=n_clusters, random_state=10)
cluster_labels = clusterer.fit_predict(X)
silhouette_avg = silhouette_score(X, cluster_labels)
print(
"For n_clusters =",
n_clusters,
"The average silhouette_score is :",
silhouette_avg,
)
结果显示分成3簇的时候效果最好
5.使用不同聚类方法
from sklearn.cluster import Birch
model = Birch(n_clusters=3)
yhat = model.fit_predict(X)
clusters = unique(yhat)
for cluster in clusters:
row_ix = where(yhat == cluster)
pyplot.scatter(X[row_ix,0],X[row_ix,1])
pyplot.show()
print("The Birch adjusted_rand_score is:",metrics.adjusted_rand_score(y,yhat))
print("The Birch davies_bouldin_score is:",metrics.davies_bouldin_score(X,yhat))
结果如下:
完整代码
#导入库
import numpy as np
from numpy import unique
from numpy import where
from matplotlib import pyplot
from sklearn.datasets import make_classification
from sklearn.preprocessing import LabelEncoder, StandardScaler
from sklearn.cluster import KMeans
from sklearn.metrics import silhouette_samples, silhouette_score
# 生成数据集
X,y = make_classification(n_samples=5000, n_features=2,n_informative=2,
n_redundant=0,n_classes=3,n_clusters_per_class=1,
random_state=1)
X = StandardScaler().fit_transform(X)
################################################################################
range_n_clusters = [2, 3, 4, 5, 6]
for n_clusters in range_n_clusters:
clusterer = KMeans(n_clusters=n_clusters, random_state=10)
cluster_labels = clusterer.fit_predict(X)
silhouette_avg = silhouette_score(X, cluster_labels)
print(
"For n_clusters =",
n_clusters,
"The average silhouette_score is :",
silhouette_avg,
)
################################################################################
model = KMeans(n_clusters=3)#聚类类别为2
yhat = model.fit_predict(X)
clusters = unique(yhat)
for cluster in clusters:
row_ix = where(yhat == cluster)
pyplot.scatter(X[row_ix,0],X[row_ix,1])
pyplot.show()
print("The KMeans Adjusted Rand-Index: %.3f" % metrics.adjusted_rand_score(y,yhat))
print("The KMeans davies bouldin index: %.3f"% metrics.davies_bouldin_score(X,yhat))
################################################################################
from sklearn.cluster import AgglomerativeClustering
model = AgglomerativeClustering(n_clusters=3)
yhat = model.fit_predict(X)
clusters = unique(yhat)
for cluster in clusters:
row_ix = where(yhat == cluster)
pyplot.scatter(X[row_ix,0],X[row_ix,1])
pyplot.show()
print("The AgglomerativeClustering adjusted_rand_score is:",metrics.adjusted_rand_score(y,yhat))
print("The AgglomerativeClustering davies_bouldin_score is:",metrics.davies_bouldin_score(X,yhat))
################################################################################
from sklearn.cluster import SpectralClustering
model = SpectralClustering(n_clusters=3)
yhat = model.fit_predict(X)
clusters = unique(yhat)
for cluster in clusters:
row_ix = where(yhat == cluster)
pyplot.scatter(X[row_ix,0],X[row_ix,1])
pyplot.show()
print("The SpectralClustering adjusted_rand_score is:",metrics.adjusted_rand_score(y,yhat))
print("The SpectralClustering davies_bouldin_score is:",metrics.davies_bouldin_score(X,yhat))
################################################################################
from sklearn.cluster import Birch
model = Birch(n_clusters=3)
yhat = model.fit_predict(X)
clusters = unique(yhat)
for cluster in clusters:
row_ix = where(yhat == cluster)
pyplot.scatter(X[row_ix,0],X[row_ix,1])
pyplot.show()
print("The Birch adjusted_rand_score is:",metrics.adjusted_rand_score(y,yhat))
print("The Birch davies_bouldin_score is:",metrics.davies_bouldin_score(X,yhat))
结果如下:
总结
以上是关于聚类方法实例的一种实现方法,供大家参考。
参考
点击阅读全文
更多推荐
活动日历
查看更多
直播时间 2025-02-26 16:00:00
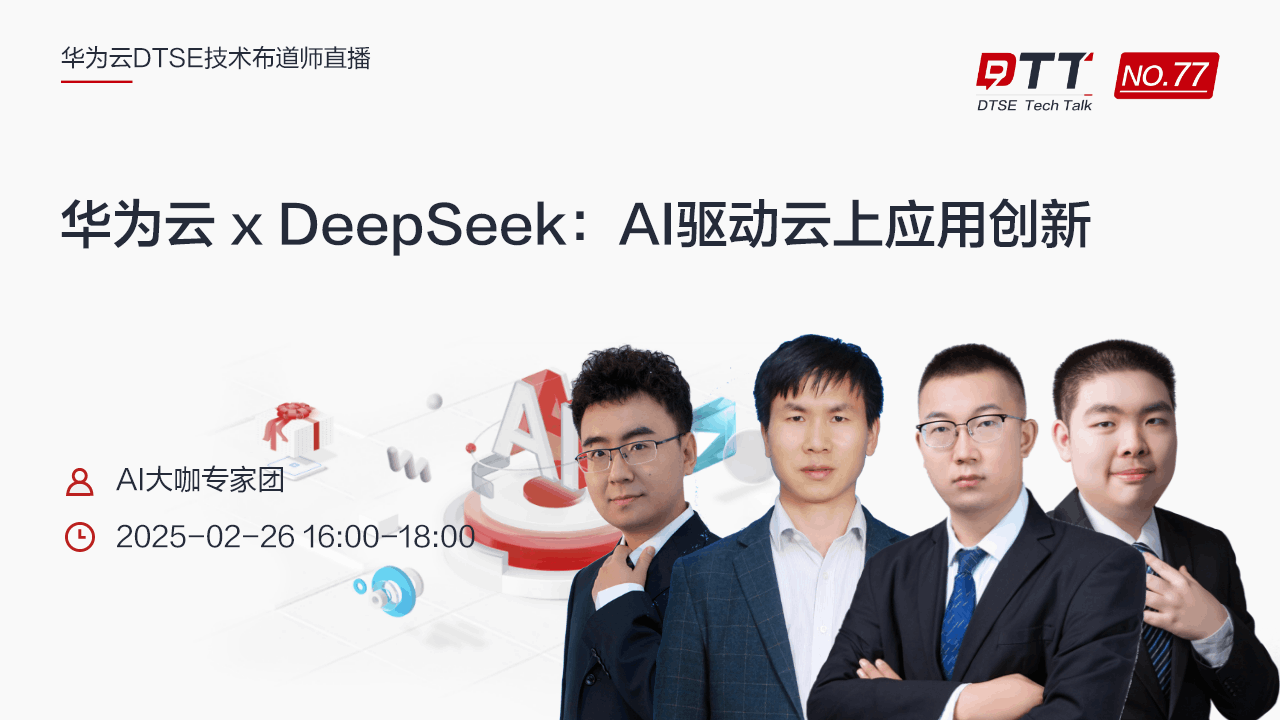

直播时间 2025-01-08 16:30:00
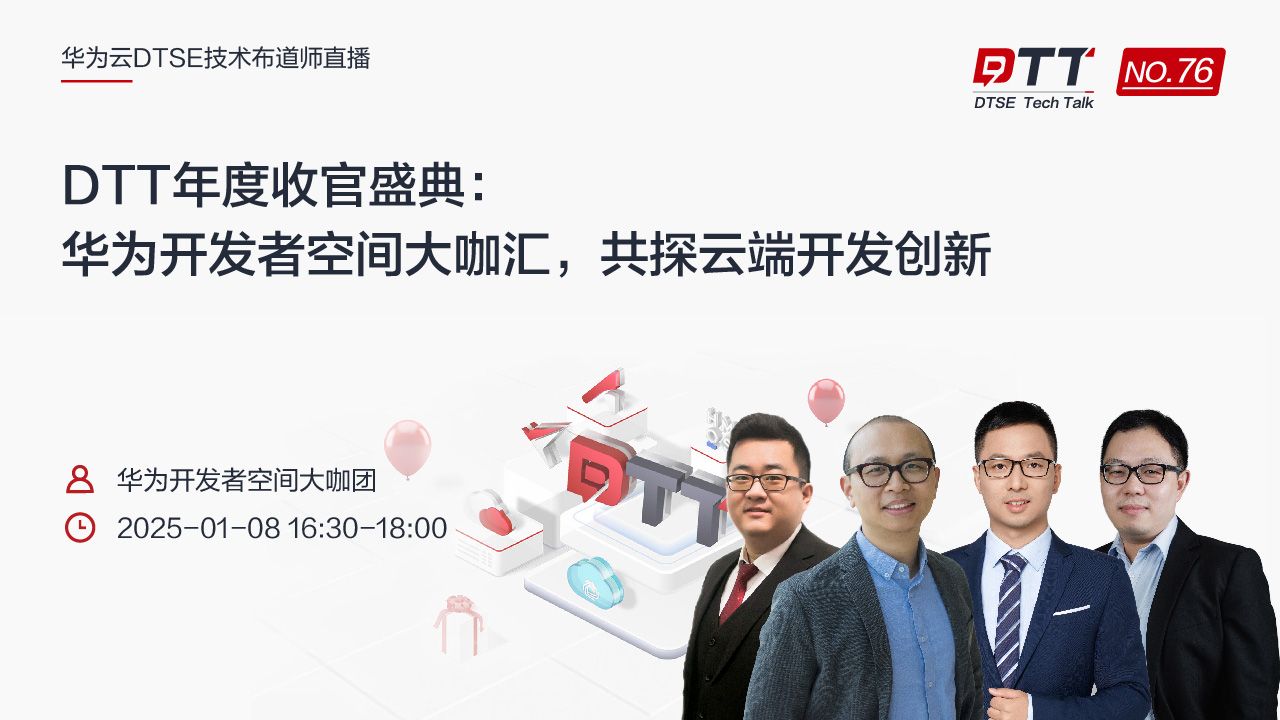

直播时间 2024-12-11 16:30:00
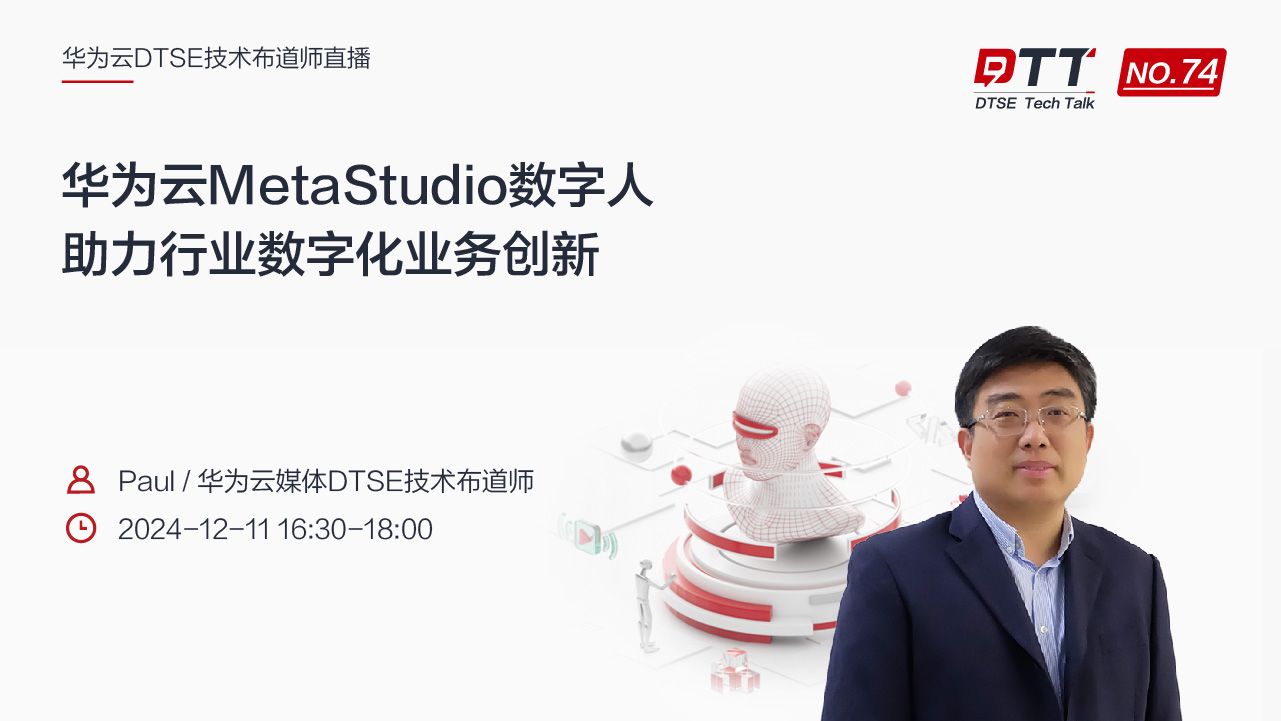

直播时间 2024-11-27 16:30:00
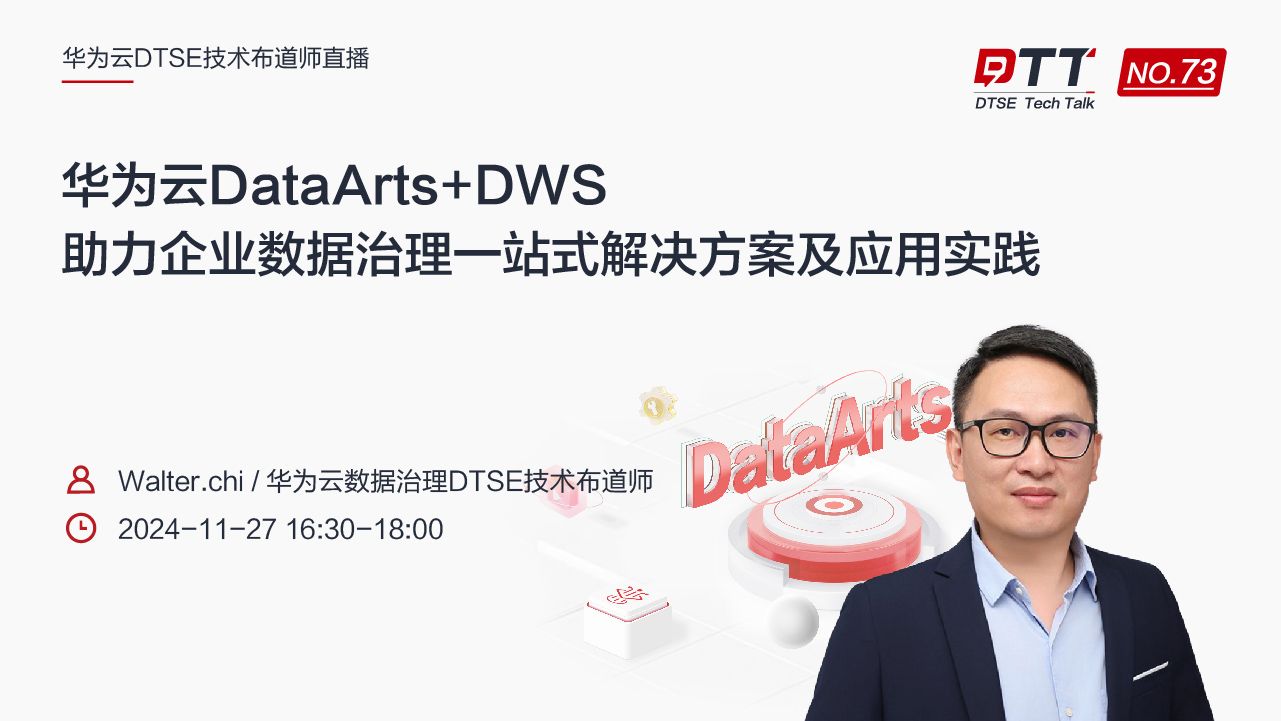

直播时间 2024-11-21 16:30:00
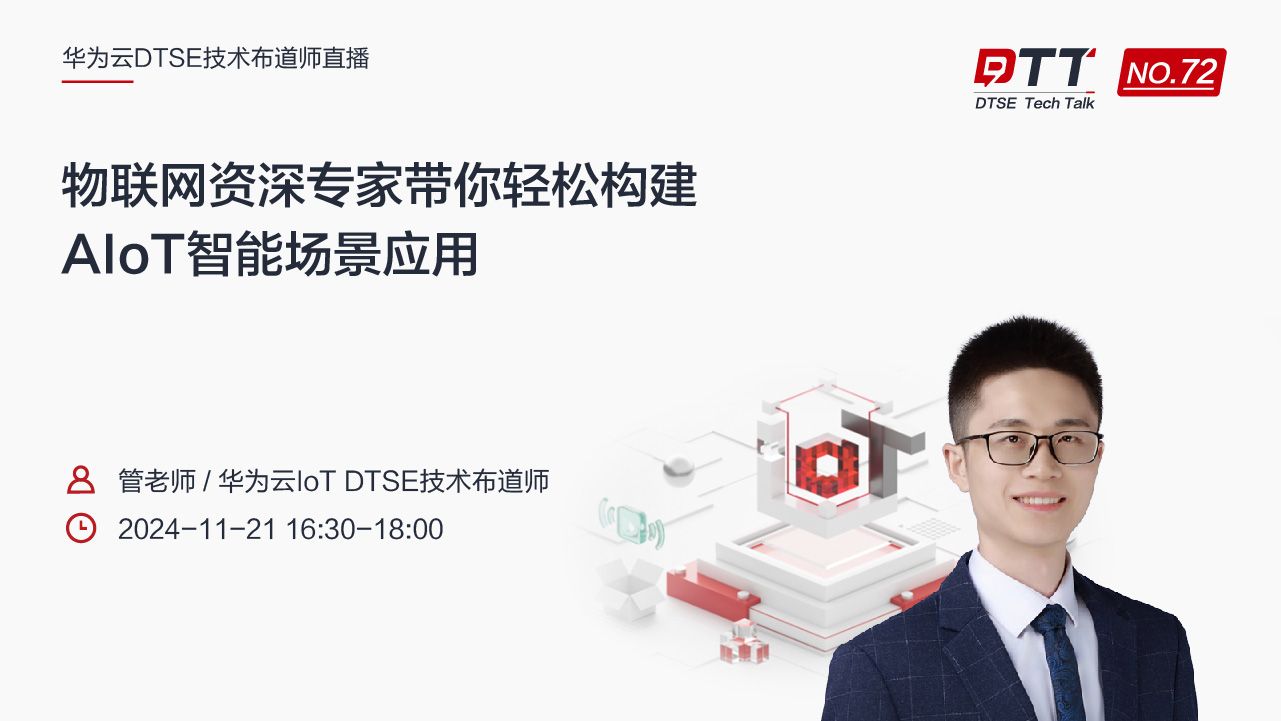

目录
所有评论(0)